Why your account scoring method has never been more important
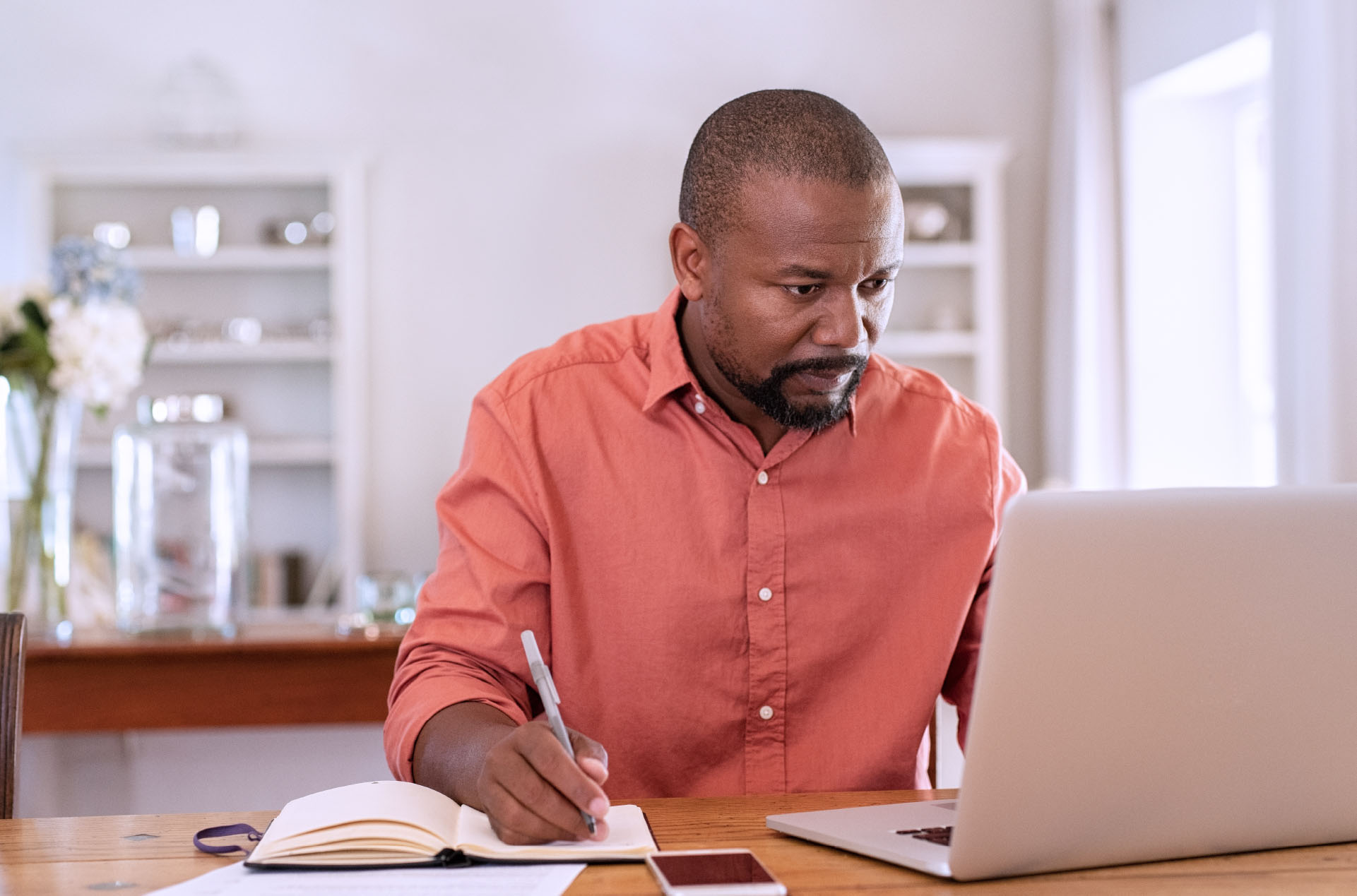
Businesses today are facing unprecedented shifts in market demand. The need for some products and services has seemingly disappeared overnight, while for essential businesses, demand has dramatically increased, even as global economic uncertainty has forced many households and businesses to reduce their spending overall. In sales, this means that you can no longer rely on past results to tell you where to focus. A better go-to-market approach for this new world begins with identifying which customer accounts are most likely to need your unique offering and prioritizing customers that are showing interest and intent to buy now.
Narrowing down a universe of possibilities
Particularly in an uncertain economy, the cost for a sales team to cover the entire universe of potential buyers is likely prohibitive, so you need an efficient process to select and prioritize within that universe. Today, most B2B market segmentation and scoring is done by combining and weighting known and observed characteristics of target accounts.
There are countless factors that a company could consider in its segmentation model: any combination of firmographics (e.g., industry, revenue, employee headcount), behaviors (e.g., search history, trends in hiring, engagement with your sales organization), or goals and desired business outcomes (e.g., entering new markets or new product releases).
Once a company has chosen its strategy and derived its scoring algorithm, it can begin to assess existing customers and potential prospects.
The account scores are vitally important in revenue planning, because they often provide a heuristic for busy sales and marketing leaders to rely on to quickly make decisions on whom to target and prioritize. But account scores and their underlying logic are also often created using limited sets of historical data captured in the customer relationship management (CRM) system that can be incomplete, inaccurate, and subjective. The result is that potential opportunities stay hidden due to bias or lack of data.
No scoring system is perfect
Even the best manual scoring efforts are prone to some mistakes because they are based on trial and error. Human error is inevitable, particularly in highly volatile market conditions, and it’s difficult to measure how often this type of error happens and the impact it may have on your business.
Let’s remove human error from the equation for a second. Say your team has developed an incredibly accurate scoring model that will return a high score 95% of the time when a company has a genuine need for your product (or the opposite, for companies where a need does not exist). You randomly select a company, run it through your model, and it returns a top score. This means that your sales team should get traction with this company 95 times out of 100, right?
Well, no.
The reality is more nuanced, because the way we should measure accuracy is not an absolute; it is relative to the incidence of some phenomenon occurring in the real world.
To illustrate, let’s say that your company is evaluating 1,000 accounts in your database for a particular cross-sell opportunity. Let’s also assume that, based on market research, the need for your new product is limited to 20% of businesses overall.
The outcomes based on those 1,000 test accounts would be:
Need | No Need | Row Total | |
---|---|---|---|
High Score | 190 | 40 | 230 |
Low Score | 10 | 760 | 770 |
Column Total | 200 | 800 | 1,000 |
One takeaway from these outcomes is that of the 230 companies that received high scores, about 83% were correct and 17% were incorrect. Not bad, but not perfect either.
A potentially more serious observation is that out of the 770 companies that came back with low scores, 1% or 10 of these were incorrect.
These should be easy wins for your sales organization—the very first place to focus when you need to shore up revenue losses—and instead they will likely be ignored.
And all this for a test with 95% accuracy.
Type I error: Chasing the wrong accounts
Perhaps the most obvious statistical error is a type I error or false positive, i.e., when a test reports that you can reject a true null hypothesis.
For sales leaders, this translates to overconfidence about a possible deal; to all the high-scoring accounts your team chases after that are really not interested in buying from you.
This type of error happens frequently, resulting in frustration for SDRs and other hunter-type sales reps. It also results in unnecessary SG&A expenses that will appear on your income statement.
Type II error: Missed opportunities
Much less discussed are type II errors or false negatives, meaning when a test fails to reject an untrue null hypothesis.
For a sales leader, this is the unturned stone that would have resulted in lucrative deals, if only you had better information.
It is the untapped market potential that everybody suspects is out there, and only the best can identify.
In any high-value sales environment, and especially in B2B sales, a single deal can mean the difference between meeting your target and falling short. Any opportunity that a sales leader can find to reduce these false negatives will help with securing and stabilizing revenue.
The next phase in account scoring
All this brings us back to account prioritization.
Most scoring models factor in variables that are correlated with good historical sales outcomes—call these “known knowns.” Some even factor in variables that are more subjective and difficult to measure but are suspected of predicting a positive outcome—i.e. “known unknowns.”
Even then, manual models miss out on thousands of variables for millions of potential customers, because you can’t seek answers to questions you never think to ask. But by identifying these “unknown unknowns” with the help of Predictive Sales Planning capabilities, sales teams can augment and improve their efforts to discover opportunities, even in a highly uncertain economy.
Say you are in a mature company that has been serving the same segments for years, but that some customers have recently had to cancel or delay orders due to an economic downturn. This is a clear case for predictive account scoring capabilities, which can help you identify promising new segments and create a plan for prioritizing those accounts with sales resources and enablement. By enriching your first-party data and expertise with a vast array of third-party market data, Predictive Sales Planning can provide powerful account targeting that helps you reduce false negatives.
Alternatively, say you are in a growing company or business unit that serves a very particular customer segment, and are looking to do more with less and drive greater profitability within that segment. Predictive account scoring capabilities from Anaplan can help you identify high-potential sub-segments and accounts within your database and reduce false positives.
Regardless of whether you are currently evaluating customers based on observable facts or harder-to-observe characteristics, having an additional estimate that leverages third-party data will help you check your assumptions and may provide you with new insights. In an unprecedented economic situation, these insights can prove critical in identifying accounts with the profile and intent to purchase today.