Characteristics of a business-friendly AI forecasting tool
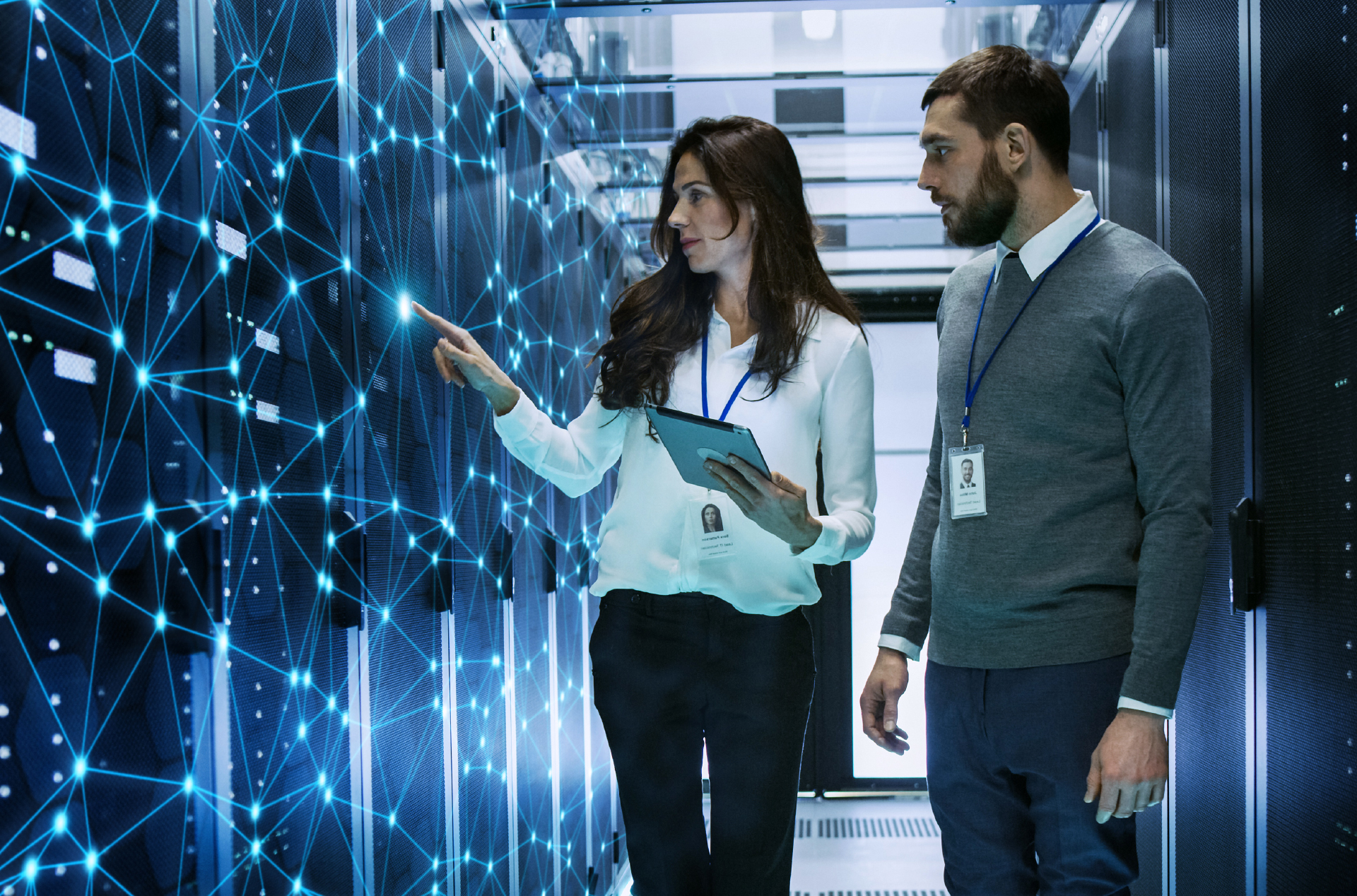
AI-based forecasting tools can be intimidating and expensive. We offer tips for finding user-friendly tools to help you make smarter predictions, faster.
This is the second blog in a three-part series that explores intelligent forecasting tools. In our first blog, we examined the role artificial intelligence (AI) and machine learning (ML) can play in intelligent forecasting and looked at some of the challenges business leaders face in adopting these technologies. In this installment, we’ll share what aspects of an intelligent planning tool can alleviate these challenges and help business users drive accurate decisions across the enterprise with AI and ML forecasting.
The previous blog detailed how business leaders are aware of the improvements AI and ML-backed forecasting can bring and know they need to find a way to predict future demand more accurately across the entire enterprise to keep pace with market changes. But they perceive ML-based forecasting as a challenging tool to leverage—and they may not be wrong.
Some intelligent forecasting tools can be intimidating. Not only can these tools be difficult for business users to access, but they can be costly and complex, requiring a considerable amount of time and resources to simply get up and running. In addition, some tools could bring an added challenge for siloed departments to share data across the business. Once a forecast is generated, some users may not understand how to interpret the insights, leaving them in the dark about taking the next best step forward.
Moreover, siloed departments that don’t have the opportunity to share and access a wide set of data can struggle to fine-tune the accuracy of their forecasts and generate insights. Even if they can input more data into forecasts, it can still be difficult to define how to use the results to take the next best step forward. These factors may indicate why only 20% of C-level executives say they leverage ML as a core part of their business.
A fresh outlook on intelligent forecasting
While the scenario mentioned above might look bleak, it certainly doesn’t have to be the case. Business leaders can harvest the benefits of an intelligent forecasting solution so long as they know what aspects to look for during the evaluation process. To gain the full benefits of a business-friendly ML-based forecasting solution, look for three key qualities: it’s accurate, accessible, and scalable.
Accurate. One of the ultimate goals of an intelligent forecasting solution is to improve the accuracy of predictions to make smarter decisions. When evaluating solutions, look for one that can evaluate multiple scenarios and derive potential future outcomes based on a wide set of historical, internal, and external data. A solution with built-in ML forecasting can rapidly test various scenarios and find correlations between them to pinpoint emerging trends quickly. A solution that can automatically select the best predictive model for each unique use case can also optimize the accuracy of forecast results.
To further boost accuracy, the solution should leverage larger data sets, bringing historical data, attributes, and business metrics together to help the ML-forecasting solution fine-tune its predictions.
Accessible. Simple deployment and an easy-to-use interface help put the power of ML in the hands of business users so that they can uncover new insights fast. A solution that doesn’t require intensive time and resources from data science teams means that business users can create and run their own forecasts, making ML-based forecasting more accessible to key stakeholders.
Plus, if the solution can automatically convert a user’s extensive set of data to a forecast engine-friendly format, businesses can eliminate tedious manual data preparation processes and save time. A solution that can simplify the integration process across both first and third-party data results in less time processing data, so teams can invest more time analyzing predictions and making more informed decisions.
Scalable. An optimal intelligent forecasting solution should also be able to scale and evolve alongside the needs of your business. A solution that’s fully integrated into your existing planning tools lets you set up and run forecasts, all in a centralized location. Users can easily leverage new data straight from the source to expand to future use cases as a business grows.
Continuous learning powered by ML helps the solution to evolve, adjust, and improve over time. This empowers users to scale their forecasts and solutions forward by years and expand horizontally across the enterprise. They can easily forecast across all lines of business, including finance, sales, supply chain, marketing, and workforce planning.
A Connected Planning approach to ML-based forecasting
An ML-based forecasting solution that is accurate, accessible, and scalable unlocks a complete end-to-end approach to intelligent forecasting that can deliver new insights and recommendations to accelerate business decisions. The ability to incorporate new datasets, pinpoint recent trends, analyze forecasts, and scale across the entire business provides business users with a complete understanding of the impact of their decisions.
It is with these capabilities and outcomes in mind that we’ve developed Anaplan PlanIQ™—a user-friendly ML-based forecasting solution that can help you drive more accurate decisions, fast. Native to the Anaplan platform and easy to use, it helps democratize ML forecasting so key stakeholders can access and understand forecasts across all lines of business. As of May 2021, PlanIQ is in Early Access with active customers.
The third and final blog in this series will feature PlanIQ use cases. We’ll look at how some customers have successfully deployed PlanIQ in their environments and explore the results they achieved.